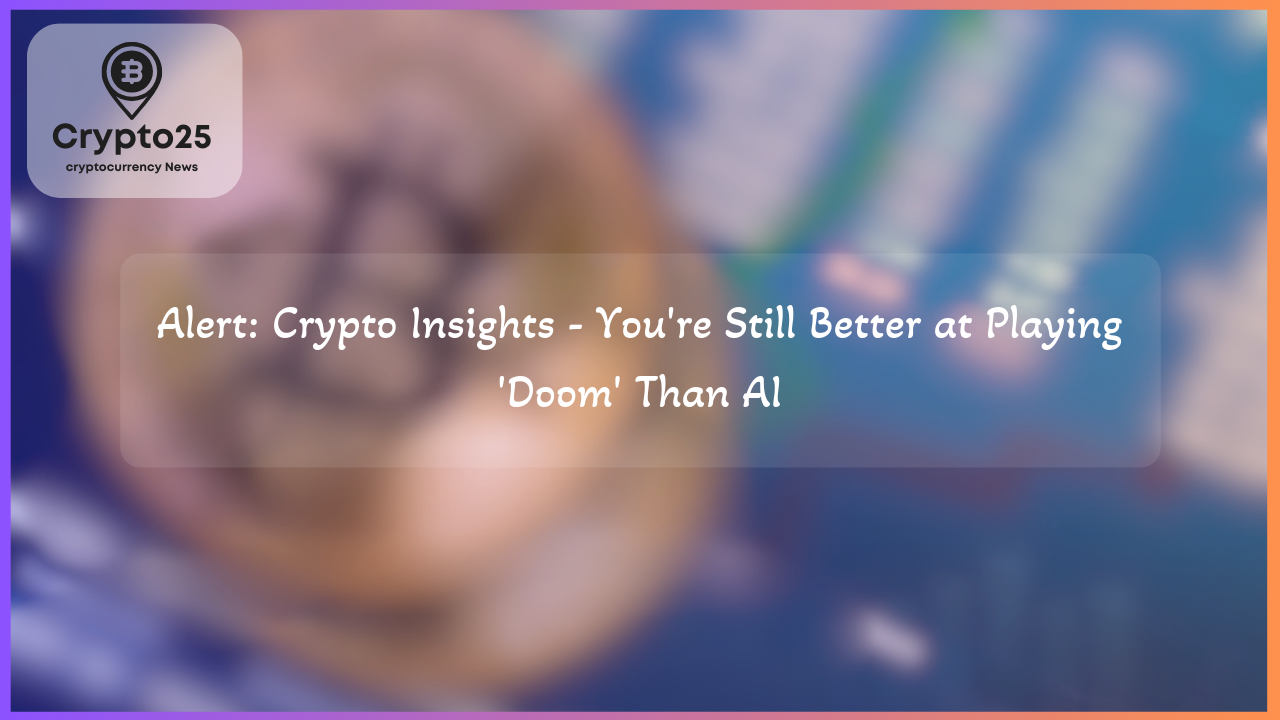
Artificial intelligence (AI) continues to redefine boundaries, yet even advanced vision-language models (VLMs) find unexpected challenges in navigating nostalgia-infused digital landscapes like classic video games. VideoGameBench, a groundbreaking benchmark for AI gaming proficiency, offers insights into how well these cutting-edge models adapt to dynamic environments. From Doom to Age of Empires, the experiment highlights the enduring complexity of human interaction in gaming, juxtaposed against machine learning’s current limitations.
### AI Models and Their Struggles with Classic Video Games
The rise of artificial intelligence has rapidly advanced technologies capable of mimicking human intelligence across a variety of fields. However, when it comes to classic video games—an unconventional yet effective testing ground for AI reasoning capabilities—even the most advanced VLMs, such as GPT-4o and Gemini 2.5 Pro, face significant hurdles. VideoGameBench, developed by AI researcher Alex Zhang, evaluates AI models using a suite of 20 popular games from the ‘90s. These games rely on visual inputs and real-time decision-making, offering an effective but challenging environment for AI systems.
Among these titles, Doom remains an iconic benchmark. Despite advancements in AI, the researchers found that most state-of-the-art VLMs struggle with tasks like timely action execution and interpreting spatial data. The problem lies in the inference latency experienced by these models. By the time the AI processes a screenshot and proposes an action, the game’s state has already changed. This lag is particularly disruptive in fast-paced action games, where quick reflexes can determine success or failure. Such experiments underline how far AI still has to go in mastering these dynamic gaming landscapes.
### The Role of Doom in AI Benchmarks
Doom, a first-person shooter (FPS) from the 1990s, has long stood as a benchmark for technological progress in gaming environments. Its enduring status is tied not only to its engaging gameplay but also to its computational design. Built on the id Tech 1 engine, Doom was intentionally optimized for minimal hardware requirements, making it a favorite tool for testing emerging technologies, from Bitcoin mining to AI algorithms.
As noted by MIT researcher Lauren Ramlan, the simplicity of Doom’s design belies the complexity of the challenges it poses to AI. Fast-paced dynamics and spatial awareness are critical in Doom, where enemy characters and environmental elements shift continuously. For instance, AI often fails to track moving enemies or navigate the intricate layouts of Doom’s levels. Such struggles reflect broader challenges inherent to VLMs, including difficulties in reasoning and understanding real-time visual data.
While simpler text-based games allow AI to rely primarily on natural language processing, visual gaming environments like Doom require nuanced spatial reasoning, multitasking, and rapid response capabilities. VideoGameBench provides a controlled framework to better understand where AI succeeds—and where it falters—in adapting to complex systems like these.
### Limitations in Current Vision-Language Models
One of the most revealing findings in VideoGameBench’s research is the inability of AI models to execute seamless in-game actions accurately. For example, models like Claude Sonnet 3.7 may be capable of identifying objectives or describing a game state, but translating this understanding into actionable in-game strategies remains a major hurdle. While testing games such as Civilization and Warcraft II, researchers observed that simple tasks, such as mouse movements or keyboard controls, frequently caused errors.
Even when the AI agent understood the desired action—such as moving a character through a room or targeting an enemy—it was unable to process these inputs effectively in real-time. This reveals a critical deficiency in how VLMs link perception with action. With slower-paced games like Pokémon, some improvements were noted. However, legacy games with dynamic environments and high interactivity rates, such as Warcraft II, further exposed the inadequate reflexes and decision-making delays in these AI systems.
In addition to these navigation issues, the AI exhibited struggles in spatial reasoning. For instance, during a Doom level, some models advanced successfully to enter the “blue room” but failed to sustain progress beyond localized areas of simplified visual data. Key in-game actions, such as shooting or dodging enemies, often became misaligned with the progression of the game state. This suggests that current AI frameworks may lack the depth of cognitive understanding necessary to thrive in environments demanding continuous multitasking and decision-making.
### The Implications of VideoGameBench on AI Development
While challenges persist, experiments like VideoGameBench contribute to the larger AI discussion by exposing the gaps that remain between human-like intelligence and machine learning. Unlike solving highly abstract mathematical proofs, performing tasks such as playing real-time strategy games or first-person shooters requires a combination of memory, spatial reasoning, and adaptability. These qualities are yet to be fully integrated into AI systems.
One significant contribution of VideoGameBench is its ability to simulate how tasks of varying complexity reveal differing levels of AI mastery. By successfully navigating Pokémon but failing Doom, the tested models demonstrated a disparity in their abilities across genres. This nuance is critical for the development of future AI systems that strive for general adaptability. Moreover, the open-source nature of the benchmark enables further exploration and iteration, inviting developers worldwide to find innovative solutions to these performance obstacles.
Title | Details |
---|---|
Market Cap | $1.2 Trillion |
By focusing on benchmarks like VideoGameBench, the AI industry gains a clearer understanding of where improvements are needed to bridge the gap between human and artificial intelligence. These developments not only apply to gaming but also hold potential for real-world applications involving decision-making systems and autonomous technologies. As the gaming community continues to intersect with AI research, VideoGameBench remains a vital tool in shaping the future of innovation in intelligent computing systems.