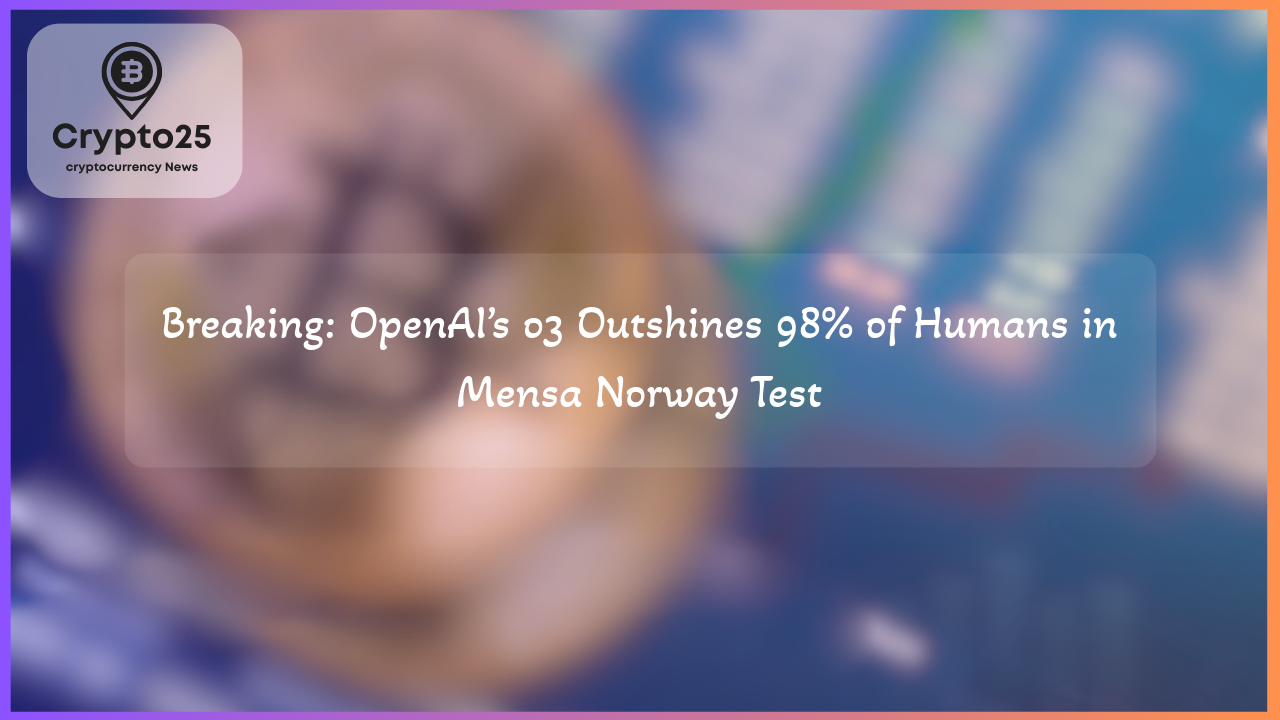
OpenAI’s latest “o3” language model has made headlines by achieving an IQ score of 136 on the Mensa Norway intelligence test—surpassing the threshold required for Mensa membership in Norway. This milestone underscores the growing supremacy of proprietary AI models in standardized intelligence benchmarks. However, the results also spark discussions about the relevance of such scores in assessing broader AI capabilities and future applications.
### OpenAI’s O3 Model Outpaces Competitors in Mensa IQ Testing
OpenAI’s “o3” model, the latest addition to the company’s “o-series,” has emerged as a new benchmark in artificial intelligence performance. Scoring 136 in the Mensa Norway test, the language model now surpasses 98% of the human population in terms of IQ, based on a standardized bell-curve distribution. Interestingly, this same model also achieved a score of 116 on a proprietary “Offline Test” administered by TrackingAI.org. These contrasting results indicate that factors such as test design and data familiarity could influence outcomes.
While proprietary tests aim to minimize exposure to prior training data, the Mensa test incorporates advanced pattern recognition and logical reasoning elements that likely align with “o3’s” enhanced architecture. These results signal a significant leap in language model development, revealing a gap between proprietary AI solutions, such as “o3,” and open-source alternatives.
Title | Details |
---|---|
Market Cap | $1.2 Trillion |
### TrackingAI’s Benchmarking Reveals Limitations Despite High Scores
To deliver consistent evaluations, TrackingAI.org employs multiple test formats, ensuring broad compatibility and reduced ambiguity in AI performance assessments. In both the Offline Test and the Mensa Norway test, models were scored based on a seven-run average, highlighting “o3’s” reliability across repetitions. However, TrackingAI’s methodology has drawn scrutiny for its opaque practices, including a lack of transparency around scoring scale conversion and prompt strategies.
The Offline Test comprises 100 pattern-recognition tasks explicitly designed to sidestep any data the AI might have encountered during training. Despite these efforts, the proprietary nature of the datasets used to fine-tune models like “o3” inherently raises questions about potential data leakage or format familiarity. Furthermore, machine advantages such as instant recall, instantaneous processing, and optimized prompting frameworks complicate comparisons with human cognition.
Even with a 136 IQ score on Mensa, experts emphasize that such benchmarks provide a narrow perspective on AI reasoning. These tests offer limited insights into real-world capabilities such as multi-turn problem-solving, long-term planning, factual accuracy, or ethical reasoning.
### Multimodal AI Models Face Performance Challenges
One of the more intriguing findings from TrackingAI’s benchmarks is the performance disparity between text-only and multimodal (text and vision) models. As seen with OpenAI’s “o1 Pro,” the text-only model scored 122 on Mensa, significantly outperforming its vision-enabled counterpart, which scored only 86. Such results suggest that the integration of multiple input modalities could introduce inefficiencies in reasoning, limiting performance under certain conditions.
However, “o3” breaks this trend by excelling in image and text analysis, a feature that sets it apart from both its predecessors and contemporary competitors. Beyond specific benchmark scores, this reinforces the argument that proprietary AI models with substantial corporate backing are likely to continue outpacing open-source systems in both research and real-world applications.
Critically, though, the broader limitations of IQ-driven assessments come into play here. While strong performance on standardized tests underlines short-context pattern recognition abilities, it tells us little about how effectively AI can generalize its reasoning or tackle unpredictable, multi-variable challenges.
### Independent Evaluations Are Shaping AI Transparency
As industry leaders like OpenAI maintain strict confidentiality over their training datasets and architectures, independent platforms like LM-Eval, GPTZero, and MLCommons are stepping in to fill the transparency gap. These evaluators conduct third-party assessments that are becoming increasingly influential in shaping norms for benchmarking AI capabilities.
Despite its success, the “o3” model raises important questions about the ethical deployment and long-term implications of such advancements. While high IQ scores on standardized tests are impressive, their relevance to general intelligence, agentic behavior, or real-world decision-making remains ambiguous. Organizations like TrackingAI.org acknowledge that their methodologies, while rigorous, still face challenges related to data leakage, evaluation validity, and reproducibility.
As more sophisticated testing methodologies emerge in tandem with accelerating model releases, the standards for measuring AI performance will likely evolve. The debate over the significance of metrics like IQ scores will continue, furthering our understanding of what defines true artificial intelligence. OpenAI and other leading companies will need to address these gaps, ensuring that superior scores translate to meaningful advancements for global users.